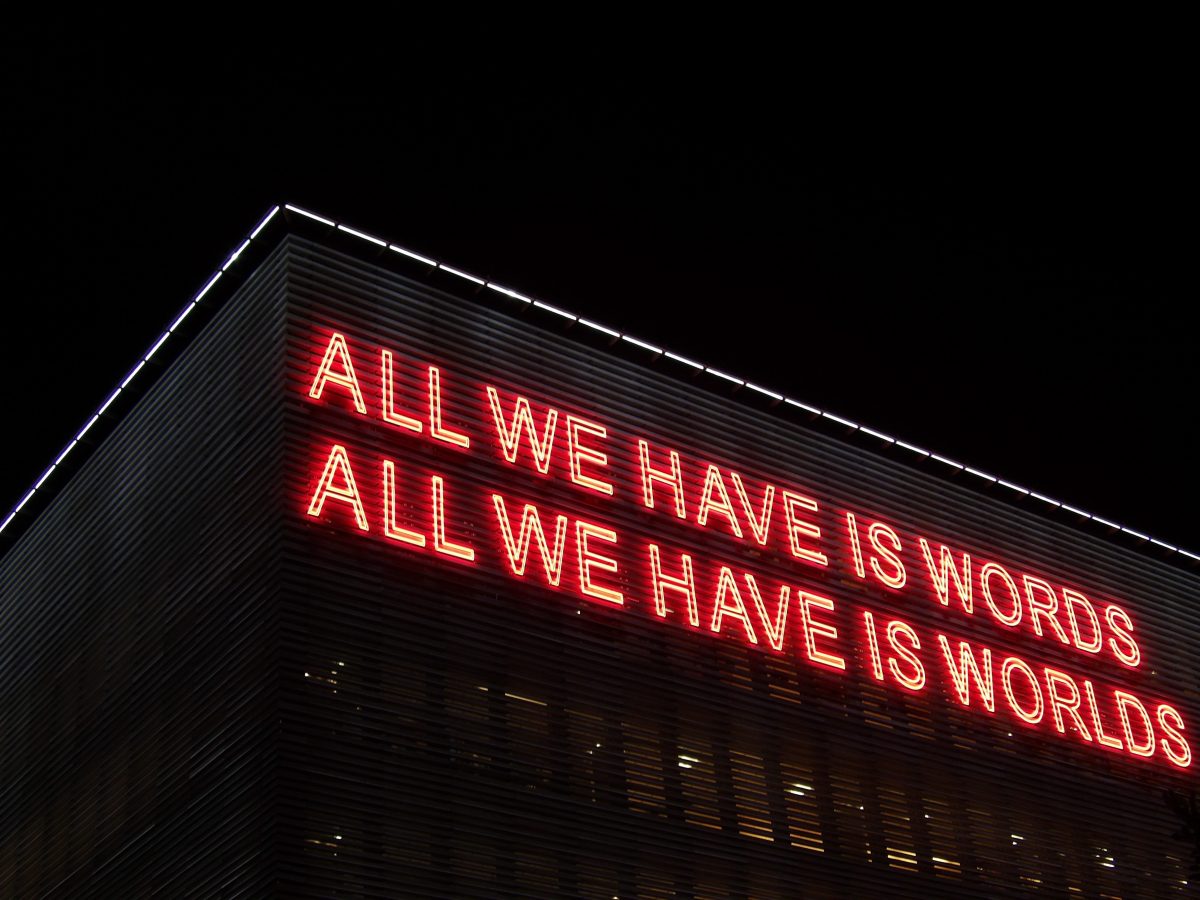
News about artificial intelligence (AI) has taken the world by storm: machine learning and related technologies are used to provide medical diagnosis, decrease business costs and even put Nicholas Cage into every Hollywood movie. However, one thing that’s yet to be tackled by AI is our human language.
But in recent years, the development of natural language processing (NLP) technologies has been swift. NLP tech is continually improving, beating academic baselines and providing better value for businesses. Already in market, we have better sentiment analysis for Voice of the Customer analytics (determining customer needs and satisfaction with current products) and chatbots for customer support or lead generation. Most of this progress relies on language modeling, a machine-learning task, but it’s controversial due to its potential for malicious uses.
So what exactly is language modeling?
A language model is an algorithm that (in most cases) does one thing: fed with existing text, it predicts what you may want to type based on the previous words. One example is Gmail Smart Compose, which predicts and offers suggestions to complete your email based on the previous emails in the conversation. To achieve this, a machine-learning model is trained on a huge body of text, such as the entire English language version of Wikipedia. By doing this, the model “learns” what words usually go together, the syntax and grammar of the language, and even some facts about the world. For example, if you type “London is the capital of…,” a good language model will generate “the UK.” This is one of the most widely used applications of machine learning: if you have a smartphone, odds are you have a language model on it to help you by generating predictive text to suggest what you may want to type next.
Today, NLP researchers are creating better language models based on neural networks, a powerful family of machine-learning models. Some of which are specifically designed to work with sequential data, making them ideal models for dealing with text which is, in effect, “sequences” of words. As a language model needs to keep track of the meaning of the text to successfully predict the right words to use, it can produce a numeric representation of texts based on its content. Computers can’t deal with text directly, so they convert it into numbers. These representations are useful for many practical tasks, like classifying a customer support request or finding relevant mentions of your products within a huge stream of social posts.
Photo credit:
Art by PNG Design on Unsplash
But you can also apply a language model to its direct task: text generation. It turns out that large neural network-based language models can generate long coherent texts that are not only grammatically, structurally and stylistically convincing, but also demonstrate real-world knowledge.
Here’s an example of how these models work. I fed a model called GPT-2 with a prompt suggesting a customer review for an antivirus product from a fake company. The model proceeded to compose a review, highlighting the non-existent product’s advantages (generated text is in italics):
I give five stars to Best Antivirus 2.0 by NotReallySecurity Company. It is fast and effective and does its job well. I recommend you all to install this application. Best Antivirus 2.0 will help you protect your computer from malware and viruses. Pros: Fast and powerful. It scans files very fast and efficiently.
Written by Transformer, transformer.huggingface.co
This is possible because the models are trained on diverse texts that include data on everything from scientific discoveries to news about cybersecurity. This has got some people worried. What if these models could be used to generate misleading or fake news, for example, arguing against public health vaccinations or trying to persuade someone to vote for a particular political group in an election?
The neural network threat of misinformation
OpenAI, a research organization, started a heated debate on the responsibility of releasing large language models with its GPT-2 model, which was shown to generate convincing fake articles about the discovery of unicorns in South America and the theft of nuclear materials in the US. The discussion continued with GROVER, created by Allen Institute for Artificial Intelligence, another model that was specifically tailored to produce realistic news text. Researchers even found that GROVER-written propaganda articles were more convincing than those written by real people.
Other applications followed. Neural network-based language models were applied to create human-like bots on Reddit, write comments about news stories, and create fake restaurant reviews, the latter application was then perfected by a CTRL model by Salesforce Research. Studies show that people had difficulty discerning machine-generated texts from real human-produced content.
The business benefits of language modeling
Despite the risks, there are many benefits for businesses and their customers. Language models power many current applications, from speech recognition and spelling correction to finding answers to questions in a knowledge base, and open up many possibilities for more intelligent language processing. Imagine a chatbot that helps people solve the problems that are currently delivered by disengaged customer support agents, or an algorithm that can write descriptions for products on a marketplace based on their characteristics. The good news? Some of these technologies are already in the market, and better models mean more natural-sounding text and more frictionless customer experience. Text-generating algorithms can also be used to automate many standardized and repetitive copywriting jobs, like the Heliograf system which ‘writes’ about results of sports events and local elections for The Washington Post. Business correspondence (imagine a smarter version of Gmail Smart Compose) could improve by using a model that automatically sets up appointments and invites the right people based on its agenda. And this is just the start.
But on the flip side, imagine an army of automated social bots spreading misinformationThey create panic around the share price of your enterprise. They spam online stores with negative reviews of your product. They overload your customer support team with plausible-looking requests, increasing costs and slowing the incident resolution time for real customers – not to mention seriously frustrating your team. These threats require better defense against automated accounts, and timely proactive detection and response to counteract disinformation campaigns.
Fortunately, the scientific community is already working hard to stop this. The authors of GROVER argue that better language models will build in fake text detectors: GROVER converts results into a classifier that can detect machine-generated news. Researchers from Harvard University and IBM used GPT-2 to create a visual tool that helps people spot machine-generated text. Scientists and academics are also working on methods to find malicious social bots based on their behavior. Meanwhile, social media giants Facebook and Twitter make removing fake accounts, which can be used to spread misinformation, a top priority.
One thing’s for sure: current progress in language modeling and NLP gives researchers and businesses powerful tools to recreate the complexities of our human language, which opens up new opportunities to support customers and create written information with ease. The tech industry needs to work hard to develop effective NLP programs and, in tandem, develop technologies to detect the real words from the fake.