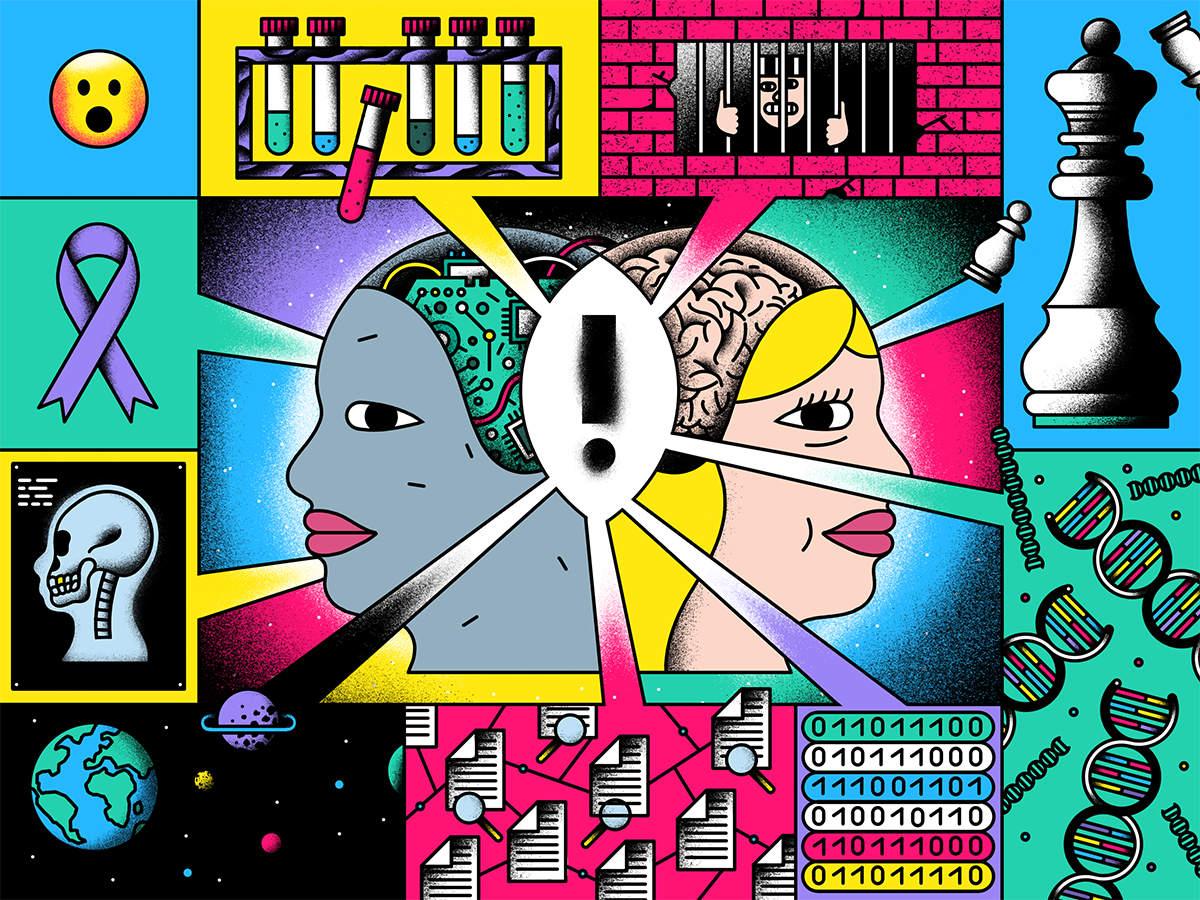
AI can mimic human thinking abilities like problem-solving and do it faster and more accurately. It’s even solved problems thought unsolvable. Google Deepmind’s AlphaFold can predict the shape amino acids will fold into, a challenge biologists have wrestled with for the past 50 years. To use the technology to speed up new drug development, Google’s parent company Alphabet recently launched Isomorphic Laboratories.
While machines outperforming the human brain may seem alarming, businesses are finding when human and machine capabilities are combined, they get the best results.
Making up for each other’s shortcomings
Researchers at Harvard Medical School and Massachusetts Institute of Technology (MIT) say their AI outperforms expert pathologists at identifying cancer in breast x-rays. AI had fewer false positives, meaning greater confidence in the diagnosis. But what’s more intriguing is what happened when combining AI and an expert pathologist. False cancer detections fell from 3.5 percent for the expert pathologist and 2.9 percent for the AI to just 0.5 percent when they evaluated the x-ray together. The AI spotted anomalies the expert pathologist didn’t and vice versa.
Designing workflows for machines and humans to work together could maximize the strengths and minimize the weaknesses of each working alone. But before we rush into re-designing organizations around human-machine teams, let’s consider the risks.
Should we be polite to AI assistants?
AI's biggest questions
We talk with Dr. Beth Singler, an expert in AI’s social, ethical, and philosophical implications.
Unintended negative consequences
AI can perpetuate biases. In the US, criminal court judges use a decision support tool, Correctional Offender Management Profiling for Alternative Sanctions (COMPAS,) to help decide whether to bail an accused. COMPAS predicts the risk the accused will offend on bail using 137 factors, such as age, gender and criminal history. It gives a one to 10 score, and if the score is five or above, most judges will remand the accused until trial.
Race is not one of the 137 factors, but a ProPublica analysis found the AI model produced race-biased results. COMPAS produced false positives, giving accused people scores of five or more who did not offend on bail, 45 percent of the time if the accused was Black versus 24 percent if they were white. The same bias showed up in predicting low risk of bail offending: Where the accused was white and offended on bail, they were much more likely to have been rated low risk than a Black accused who offended on bail.
This bias came from the data used to train the AI model. There was bias in choosing data to use or in the data itself, affected by prejudice in past judge’s decisions. Over-reliance on sophisticated algorithms that perpetuate prejudice should concern us.
Using AI responsibly
Research institutes, governments and corporations have started thinking about what it means to use AI ethically.
Most leading organizations have, or are working on, an ethical or responsible AI policy. One of the most-cited principles is ‘explainability,’ meaning a human can explain how an AI model reached its conclusion.
With some forms of AI, you can see what the algorithm is doing and the data it generates. But it’s near impossible for humans to understand what’s happening in the billions of numbers crunched by applications that use large neural networks – algorithms mimicking how the human brain works. Experts are developing tools to help extract information from these models, and there are efforts underway to develop more explainable AI models.
Meanwhile, organizations are adopting measures to govern their AI capabilities, following Google’s lead with their AI ‘model cards’ showing compliance with ethical approaches to choosing and verifying AI training data and validating the AI model. They’ve kept sight of a simple truth: An AI system’s human operator is responsible for explaining why they used the model in a certain way to support their decisions.
AI and human superpowers combined
AI’s ability to outcompete humans at some tasks is in its data processing scale and speed. It can assess many alternative paths at the same time. Testing many hypotheses can yield successful strategies that would be too expensive, time-consuming or dangerous for humans to experiment with. But machines do not yet have a sense of right or wrong, nor imagination to hypothesize about things that haven’t happened.
How should we make best use of AI and humans working together while reducing the risk of unintended consequences? Nicky Case’s prizewinning essay in the Journal of Design and Science proposes:
Computers are good at deciding the best answers; humans are good at deciding the best questions.
Consider how humans process information. We take in data from our senses, add structure to create information, then link that information with experience to create knowledge. From knowledge of the situation, we determine what action to take in the form of wisdom. AI can do most of the heavy lifting of processing data and information, so humans can spend more time gathering knowledge and proposing courses of action that might find better outcomes.
The relationship between AI and humans is in its infancy. We don’t yet know how it may evolve. There are fears machines will replace us in many work contexts, from the production line to radiology. Every job that exists today will be affected – positively and negatively – by AI. Leaders and engineers must understand the impact their decisions about AI will have on society.